Moving in Space and Time
Deep-learning-driven large scale information extraction from historical maps and high resolution aerial images
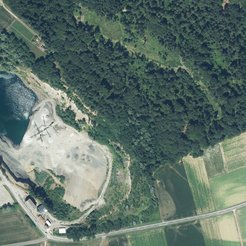
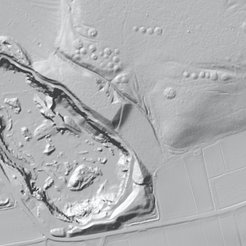
Statistical series, scientific journals and maps, especially cadastral map data from the long 19th century, bear incredibly valuable information about human environments, landscapes, and infrastructures. This information is of utmost value to geoanthropology, as it allows the detailed reconstruction of processes, causalities, and complex human-nonhuman-interactions in the context of the Great Acceleration.
I explore methods and approaches to reconstruct the surface of the Earth as it has been used by humans in a historical dimension. Therefore I need to find ways to extract the relevant information stored in the digital representations of maps, statistics, and textual descriptions in a structured way, in order to contribute to a better understanding of the ways in which human interactions have had an impact on landscapes, vegetations, and further relevant features of the surface of the Earth.
To extract, process, and visualize the relevant data, I use different procedures from the field of machine learning, from natural language processing to image detection and segmentation. In order to relate the results of this work to the present, I further use high-resolution aerial imagery including LiDAR data, corpora of satellite images and further serial data.
The Franciscean Cadastre serves as a starting point for this endeavor. It was produced for the territories claimed by the Habsburg Empire between 1817 and 1861, covering todays Austria, Slovenia, Croatia, Czech Republic, Slovakia, Hungary, parts of Romania, Poland, Northern Italy, Serbia and Montenegro and a total surface of c. 670.000km2. The information it contains on settlements and infrastructure and land use are complemented by comprehensive statistical data and further descriptions, extracted from several digital repositories. The data were raised in census operations including livestock census, scientific inquiries, and further administrative operations.
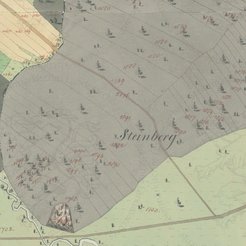
My current work is funded by the FWF (project reference PAT1763723), and focusses on the upscale of the extraction of information on infrastructure and past land use from historical cadastral maps. Extracted information is then integrated into a digital terrain model, where it can be visualized and analyzed in a time series.
Latest publications
2024: [in print with DHQ] GÖDERLE, Wolfgang (with Christian MACHER, Katrin MAUTHNER, Oliver PIMAS, Fabian RAMPETSREITER): Deep Learning for Historical Cadastral Maps and Satellite Imagery Analysis: Insights from Styria's Franciscean Cadastre. Forthcoming with Digital Humanities Quarterly in 03/2024.
2024: [accepted by IJDL, preprint available] GÖDERLE, Wolfgang (with David FLEISCHHACKER and Roman KERN): Improving OCR Quality in 19th Century Historical Documents Using a Combined Machine Learning Based Approach. Preprint: https://doi.org/10.48550/arXiv.2401.07787
2024: [preprint] GÖDERLE, Wolfgang (mit Christian MACHER, Katrin MAUTHNER, Oliver PIMAS, Fabian RAMPETSREITER): AI-driven Structure Detection and Information Extraction from Historical Cadastral Maps (Early 19th Century Franciscean Cadastre in the Province of Styria) and Current High-resolution Satellite and Aerial Imagery for Remote Sensing. Preprint: https://doi.org/10.48550/arXiv.2312.07560